Diamond Solutions Releases Rapsodo 1.0/2.0 Pitching Data Guides for HS
Diamond Solutions Releases Rapsodo 1.0/2.0 Pitching Data Guides for HS
Diamond Solutions Nate Walker releases Rapsodo 1.0/2.0 Pitching Data Guides for high school and college players.
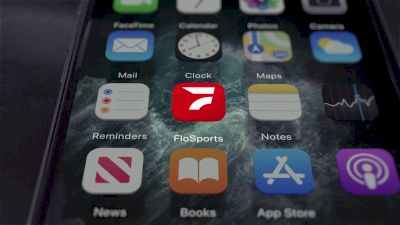
- Auto
- 1080
- 720
- 480
- 360
- 134
Unlock this article, live events, and more with a subscription!
Already a subscriber? Log In
Nate Walker is currently the Founder and President of Diamond Solutions, an analytics consulting firm that assists softball and baseball organizations of all levels with their player development and evaluation needs. Prior to starting Diamond Solutions, Nate worked for two Major League Baseball front offices where he served as a liaison to the Major League coaching staff, assisting them with game preparation, in-game strategy, player acquisition, and statistical research.
Over the past two years, I have been traveling the country collecting data on a normal distribution of talent to figure out how ball-flight data (i.e., Rapsodo) can be successfully applied to softball player evaluation and player development.
After tens of thousands of pitches and nearly a hundred thousand miles in travel, I can gratefully and proudly say we have assessed players ranging from three Olympic teams, WCWS champions, WCWS participants, Super Regional and Regional teams, Power Five schools, mid-major programs, and hundreds of travel teams with varying levels of high school talent. Before starting my company, Diamond Solutions, I worked in two MLB Front Offices and saw firsthand how valuable this information was for both management and the players. As a result of that experience, I sought to continue to share that value with as many softball organizations as possible in order to help grow the game’s player evaluation and development methods.
Now that the sports landscape has drastically changed these past few months, there has been an added emphasis on developing and evaluating players remotely, leading to a greater reliance on data, technology, and video. Given how significant these changes are for softball, I felt it would be beneficial to release my Rapsodo pitching data guides (High School Recruiting and College) so coaches and players have a better understanding of how each metric varies.
Please keep in mind that these charts are not the sole determinant of whether or not a pitch/pitcher is effective. Instead, they are meant to describe how a metric’s normal range varies within our sample of pitchers (see “How Should I Use the Charts” question below for further explanation). To view the data guides, please click the hyperlinks below.
Additionally, each data guide has documentation that contains notes on all the data ranges. There is also a FAQs document that answers some of the most popular questions I receive on this information. If you do not want to read all of the notes/FAQs (highly recommended for better understanding) on the data guides, I have answered the three most important questions below.
How Should You Use The Charts?
As I mentioned above, these charts are more of a descriptive tool, not a predictive one. The primary purpose of the charts is to get a brief overview of where a pitcher’s metrics lie within a specific range.
For example, if Pitcher A has a dropball that has -8 inches of vertical break, that means the pitch has above-average sink. It does not mean the pitch will produce above-average results, but it is definitely worth knowing this characteristic to determine how the pitcher can develop a more complete repertoire that will ultimately produce above-average or better results.
Remember, all MLB teams have access to the same ball-flight data but have different approaches when it comes to player evaluation and development, ultimately proving that the proper analysis of all the metrics is the true competitive edge, not just having access to a chart that describes whether a number is high or low on a scale. The predictive portion of the analysis involves using all of the information displayed on the chart to determine if a pitcher has or is close to an optimal velocity/spin/movement combination.
For example, there are pitchers with low/average spin rates that have some of the best strikeout numbers of all-time, while there are other pitchers with above-average spin rates that struggle to make it through a lineup twice. By understanding how the different combinations of velocity, spin, and movement play off one another, you would be able to explain why pitchers with below-average characteristics can produce above-average results and vice-versa.
To summarize, the predictive component of any analysis is the competitive advantage when incorporating data into your program, however, you can’t get good at prediction or making decisions with the data until you understand how to properly describe each pitch, which is the entire purpose of sharing these charts.
What Are The Important Differences Between The High School And College Scales?
The most obvious difference you should notice between the two scales is the format. The college scales use a more traditional range format while the high school scales use “green” and “red” zones. The purpose of the green/red zones format for high school players is to focus more on quickly identifying characteristics on the extreme sides of the bell curve while not being overly harsh on the players whose metrics are mostly in the middle. Green signifies the metric is above-average on a collegiate scale, and red represents the metric is below-average on a high school scale.
When deciding how to evaluate each metric, the first step is to determine which metrics are more of a function of strength/age and which are more of a function of skill. We identify velocity and spin as strength/age-based metrics and vertical and horizontal break as skill-based metrics.
For spin and velocity, there is a significant difference between the high school and college samples, and those differences are reflected in the data scales. When evaluating spin rates on younger pitchers, we prefer to use the normalized spin rate (spin rate divided by velocity) because it gives us a better understanding of what a low, average, above-average and high spin should be when controlling for velocity (i.e., for every 1 MPH, the pitcher produces 17 RPMs of spin).
This approach makes it easier for us to compare a high school pitcher’s ability to spin a ball to a college pitcher despite the significant velocity differential. If the high school pitcher’s raw spin rates were already above-average on a college scale, she would be rewarded for that on our reports.
For vertical and horizontal break, the average difference in movement between high school and college pitchers is not that large (about 1-2 inches), which is why the below-average/above-average cutoffs remain relatively similar.
The most notable difference is that the vertical break red zone in the high school scale is more forgiving than the “Low” vertical break range for the college scale. Please note that when drawing cutoffs for the “red” zone of the movement chart, we took into account how the pitch-type should move and did not overly rely on how the pitcher classified the pitch.
For example, you could make the argument that the lower range of riseball vertical break could be extended into the -2/-3 range, but we would argue that a pitch with -2/-3 inches of sink is technically a fastball, therefore the start of the “red” zone for vertical break should be adjusted to -1. I also want to note that even though the overall difference in movement between high school and college pitchers is not significant from a pure averages standpoint, there is still a notable difference when you analyze how the pitches move relative to one another, specifically with regards to the better college pitchers.
When you track a normal distribution of high school talent, you are still going to see the down-ballers, up-ballers, and everything in between just like you would in college, so when you analyze the samples, the college and high school movement numbers will be somewhat similar. But let it be known that there are noticeable movement differences with the more advanced college pitchers, and those differences become more apparent when we take into account the quality of the velocity/spin/movement combination as a whole.
How Do You Identify Data Errors?
It is important to note that no matter how much money you spend on a ball-tracking device, they will always be prone to data errors. It is an essential responsibility of the data operator to identify data errors, or else you run the risk of providing a player or coach with an inaccurate analysis.
The easiest way to identify data errors is to study the different ranges for each metric, particularly total spin, vertical break, and horizontal break so that the machine operator can quickly identify whether or not a data reading consistently falls out of a metric’s expected range.
For example, if a pitcher throws a riseball that has 8 inches of positive vertical break, but the majority of the riseball readings are between 0-2, that 8-inch reading is not an exceptionally good pitch, it is a data error. If you are on the fence of whether or not a data reading is legitimate, have the pitcher keep throwing the pitch until you get a better sense of her true data range. If you are still uncertain, play it conservative and delete what appears to be any extreme data point. In small sample situations like bullpens, a conservative approach is always better because you do not want the outlier measurements significantly affecting the averages/medians.
Questions?
Feel free to reach out to me via email (nhwalker34@gmail.com) or Twitter (@DomiNate34).